In conversation with the ICTU Statistics team #StatsCI #lovingclinicaltrials
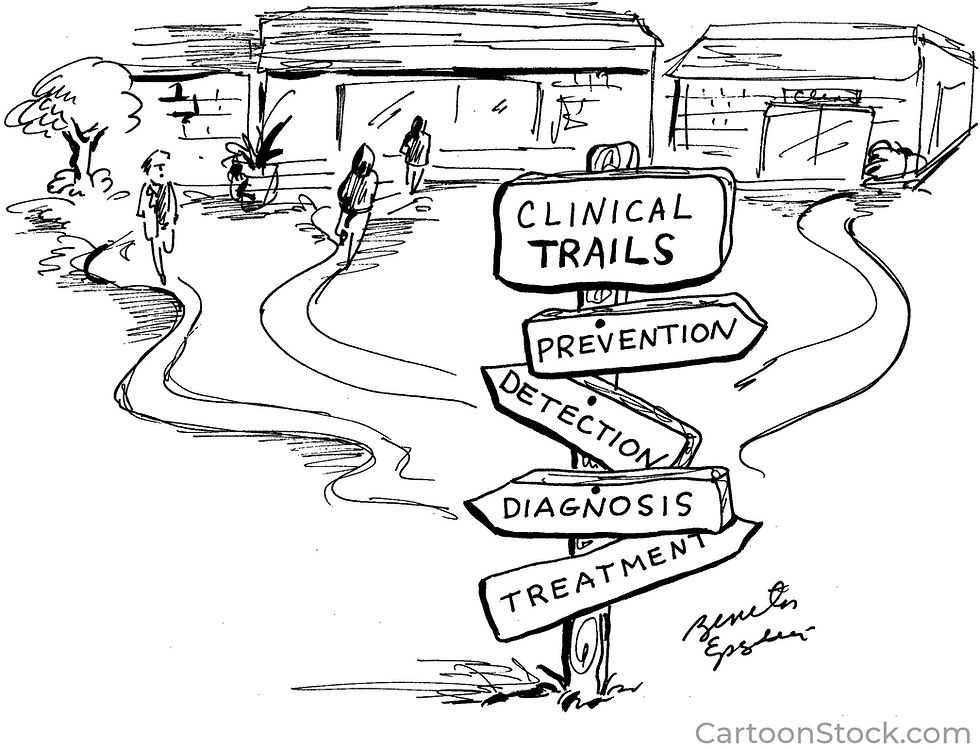
In celebration of clinical trials day, our statistics team got together to chat about some of the facts we think you may find interesting about trials and what trials mean to us...
A sample size calculation (to work out the number of patients to include in a trial) actually takes quite a long time. This is because there are many assumptions that need to be estimated and justified which takes carefully thought and time. This is to ensure enough patients will be included to identify whether the treatment being tested works, whilst not exposing too many patients to new treatments with lesser-known safety profiles. It is often perceived that this is a "quick” calculation, but a “quick sample size calculation” is only possible if you know and have decided on everything about a trial. Rarely is this the case…in fact we are not sure we have ever come across the situation where the trialists have this all in hand prior to a discussion with us. With new trial designs, simulations are often required, and this can take weeks to perform.

Analysis involves more than simply pushing a button or running a single line of code. We write detailed statistical analysis plans before we look at trial data and these specify every model we will fit and how, as well as how we will handle missing data in the analysis and many other aspects. This is to avoid bias from selectively reporting results that provide the most favourable conclusions after running multiple different analyses. Constructing datasets for analysis from large complex databases takes a while. Cleaning data, constructing the statistical code and getting this validated by a second statistician to have confidence in our results can often take months. Preparations begin during a trial but there is still a lot to do when we get the final clean and locked dataset.
There is a NO single correct way to analyse a trial. Different statisticians will use different methods and make different assumptions and that will mean the results could slightly differ depending on who designed the analysis plan. That is another reason why it's so important that we write a detailed statistical analysis plan prior to looking at the data. We make sure we have approval from the Chief Investigator as well as oversight committees (Trial Steering Committee, Data Monitoring Committee).
Trials are difficult! Many researchers external to the field convey the perception that the statistical methodology is easy in trials, and this involves little more than recruiting two or more groups and comparing outcomes between the groups through unadjusted hypothesis tests or a quick regression model. This is simply not true, we have come a long way in trials research and the trial statistician needs to have expertise in a whole array of statistical techniques. There is currently fast momentum to advance trial design and analysis and it’s really exciting (if sometimes challenging!) to learn and implement newly emerging methods, such as adaptive trial designs where key features of the trial design (often treatment arms or sample size) are changed party way through the study.
There are also regulatory considerations and multiple guidelines being developed
and updated all the time. Trial methods have advanced and become specialised so
much that not every statistician will be able to do every trial.
Some novel trials with multiple treatment group and adaptive design features require more than one trial statistician. Such trials often require regular analyses during the trial to determine whether the trial should be changed in a particular manner. This all takes more time than traditional parallel group trials and can also require complicated statistics. This may require statisticians with particular expertise, in additional to general trial statisticians.
It is hard to get a trial published where the intervention does not show a benefit, even though the research question is deemed an important one! It can even be hard sometimes to get a trial result published if it shows benefit as journals often want to only publish questions that they deem are cutting edge. This is problematic, as not publishing a trials' results means other researchers wont know about it and may undertake a similar trial, resulting in wasted resource. It can also introduce bias in meta-analysis providing false promise for ineffective treatments as not all the evidence is taken into account (reporting bias). The consequence of which can be patients receiving ineffective treatments.
RA Fisher - “To consult the statistician after an experiment is finished is often merely to ask him to conduct a post mortem examination. He can perhaps say what the experiment died of.” This quote captures how crucial it is to get trial design right to ensure the answer to the question of interest will be obtained. Trials need to be carefully designed to ensure the right data is collected, and to ensure the analysis is of relevance.
The importance of patient safety and maintenance of rigour is always paramount. Throughout a trial patient safety data is continuously reported and assessed by oversight committees. This does mean clinical trials must follow some heavy duty and sometimes overcomplex processes, which can hinder the timeliness of conducting research and getting research results into practice.
The rigor of trials and increasing regulatory burden means that trials have become more expensive to conduct over the years. They also take a long time, feasibility trials are often 3 or more, and for later phase trial it can typically take between 5 to 7 years to conduct. The pandemic demonstrated the success that embedding trials in routine clinical practice can have, and the regulators excelled with a speedy review of data. It would be good to see a change of practice to enable us to conduct all trials faster. The example trials in the pandemic suggest we would benefit greatly from moving to embedding trials more in routine clinical practise.
A robust and validated database is vital as the data collected in the trial is central to answering the question of interest. But constructing a database, keeping it secure, and transferring data can be difficult, expensive, and slow. It can be the cause frustration for the different team members. There is a balance between collecting extra useful data in a trial and the burden and cost of collecting data ‘just in case’, there seems no short cut to getting this right for differing trial teams.
Statisticians can be trial participants too! We discovered that members of our team have not only analysed trials, but been participants in different trials. In can be highly rewarding to be a participant in a trial and know that your data is helping to find new and better treatments to shape the future medical care of others.
Most clinical trials are referred to by acronyms. Some of the best ones we have seen

include the PROSECO trial (A study of the immune response to COVID-19 vaccination in people with lymphoma) and the SuDDICU (Selective Decontamination of the Digestive Tract in Intensive Care Unit Patients). It's surprising how long it takes to find a good acronym! But getting suitable branding and imagery is important for generating publicity to help the recruitment of patients.
We have seen celebrity endorsement increase the recruitment of trial patients. In a recent trial in prostate cancer, a single tweet by the actor/broadcaster/comedian Steven Fry generated a huge uplift in the number of patients wanting to join the trial. However, care must be taken when using celebratory endorsement to ensure it does not unintentionally impact the characteristics of the patients included in the trial. It is important that trials recruit a wide selection of patients (as suitable for the medical area under investigation) so that results will apply to the population of interest.
So, why do we love working in trials?
Fundamentally trials are the only robust way to reliably establish causal inference allowing us to answer important medical research being part of a multi-disciplinary team achieving that (without having to get our hands physically dirty) is highly rewarding. The existence of trials underpins evidence-based medicine and helps doctors to know what works and what does not wor
The highly rigorous procedures that support full transparency of the research process, such as the full intentions of a trial being documented up front in a protocol, deciding on what measures should be prospectively collected and building a reliable system to collect data, pre-specifying the analysis, endpoints and comparisons in advance (and many more!) mean that well-conducted trials are at a far lower risk of bias compared to other studies, another reason why they are highly regarded. The ethos of these good practice processes in trials are starting to be adopted in other observational studies- they have been called target trials, and this is really heartening to see.
Some people may think trials analysis is straightforward and would be repetitive for statisticians working in the field- maybe it used to be in the early days when the principles of trials methods were being established. But long gone are the days of unadjusted analysis using t-tests and chi-squared, ignoring missing data and clustering, and not taking account of multiple looks or multiple comparisons! There have also been advances in analysis to determine how an intervention works not just why. The designs are also becoming more adventures and complex, so as statisticians we are constantly updating and learning new skills – it’s certainly not boring or routine!
For statisticians, our involvement is needed at every stage, from a clinical investigators early novel idea, to helping shape the trial design, securing funding, conduct, and final publication. One of the most rewarding parts is the relationships we get to establish with highly skilled clinical teams whom we will work with over many years. Feeling influential in the process is important to us and one that is rewarding as we know we can help to deliver a high-quality study.
Ultimately working in trials research feels a great way to contribute to society- it is helping to provide the highest quality evidence to inform treatment and improve patient outcomes – what is not to love! Happy Clinical Trials Day 2022!

Victoria Cornelius, Suzie Cro, Emanuela Falaschetti, Jack Elkes, Ellie Van Vogt,
Consuelo Nohpal de la Rosa, Elli Bourmpaki, Leila Janani
Comentarios